Part 14 of The series where I interview my heroes.
Index and about the series“Interviews with ML Heroes”
You can find me on twitter @bhutanisanyam1
In this very interview, I’m super excited talking to another great kaggler: The Discussions grandmaster: (kaggle: @tunguz, ranked #3), Kernels (Ranked #10) and Competitions Master (Ranked #23): Dr. Bojan Tunguz
Dr. Bojan Tunguz holds a Ph.D. in Applied Physics from the University of Illinois and a masters in Physics from Stanford University.
He is currently working as a Data Scientist at H2o.ai, before H2o.ai he had worked at Figure as a Data Scientist and at ZestFinance as a Machine Learning Modeler.
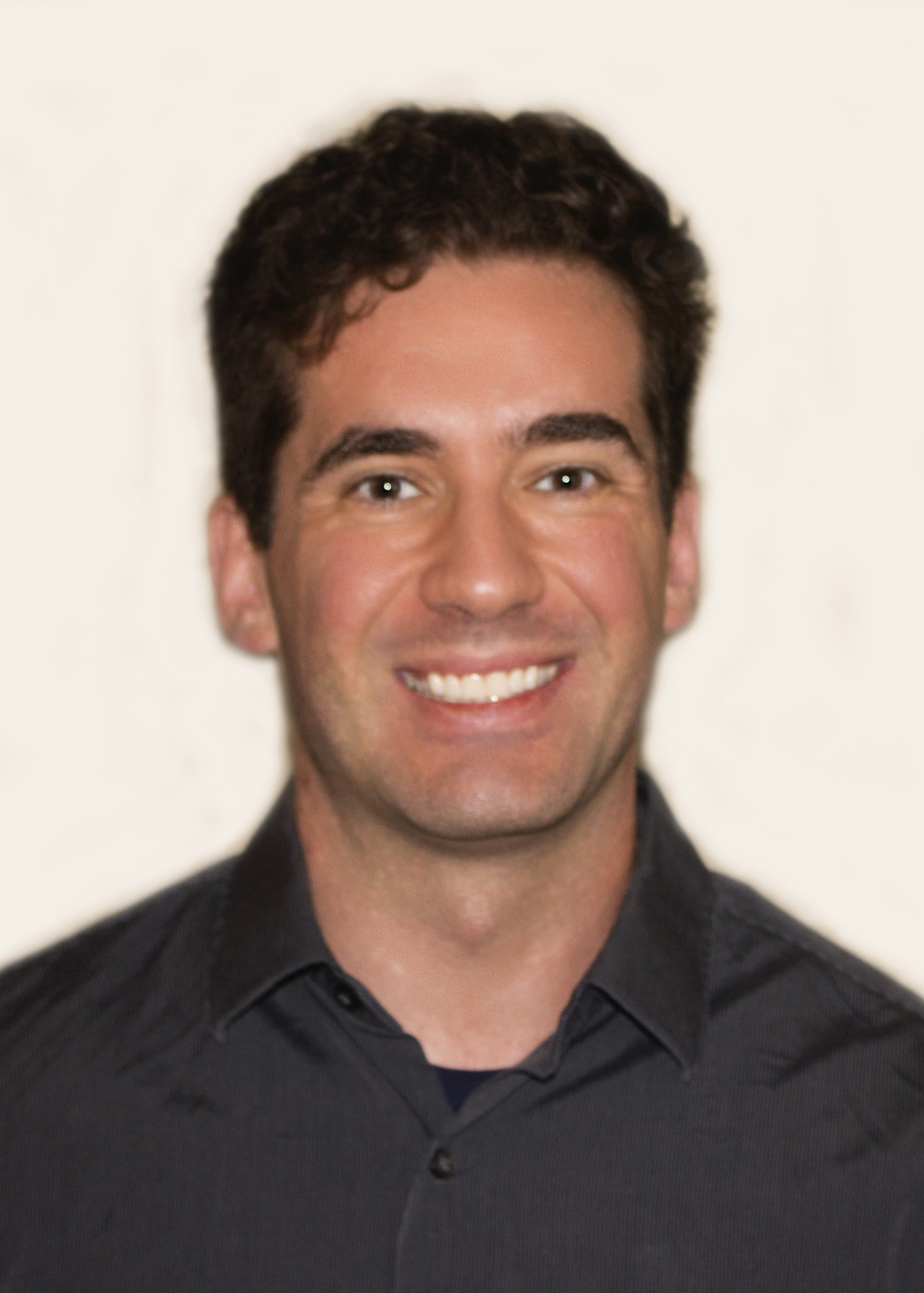
About the Series:
I have very recently started making some progress with my Self-Taught Machine Learning Journey. But to be honest, it wouldn’t be possible at all without the amazing community online and the great people that have helped me.
In this Series of Blog Posts, I talk with People that have really inspired me and whom I look up to as my role-models.
The motivation behind doing this is, you might see some patterns and hopefully you’d be able to learn from the amazing people that I have had the chance of learning from.
Sanyam Bhutani: Hello Grandmaster, Thank you for taking the time to do this.
Dr. Bojan Tunguz: My pleasure, it’s great to connect with you.
Sanyam Bhutani: You’re on the Top 3 of the Discussions leaderboard today and in the Top 10 and 25 for Kernels and Competitions. (At the time of the interview)
You have a background in Physics. How did you get interested in Machine Learning and in kaggle?
Dr. Bojan Tunguz: The only viable career option for someone with a background in Theoretical Physics is an academic job. However, over the past few decades, academic jobs have for all the practical purposes dried up. A combination of unappetizing career options in Physics, personal considerations, and other factors spurred me on to look into the alternatives. Fortunately, I’ve always had a very broad interest in a variety of intellectual pursuits, and had almost accidentally stumbled upon a few high-quality online Machine Learning courses. After a while, I also started competing on Kaggle and quickly realized that the challenges, insights, and the resources that Kaggle provided far exceeded anything that I had previously encountered in any educational environment, online or offline.
Sanyam Bhutani: You’ve recently joined as a Data Scientist at H2o.ai and have been working as a consultant during the past few years.
Where does kaggle come in the picture? Is it related to your other projects?
Dr. Bojan Tunguz: Kaggle has been the single most influential factor in my career as a Data Scientist thus far. Actually, prior to joining H2O, I had worked for a couple of other tech startups, and for both of those jobs, my success on Kaggle had been one of the crucial considerations in getting those jobs.
At H2O we take Kaggle success one step further. Our most advanced product, DriverlessAI, distills the collective wisdom of several Kaggle Grandmasters that we have worked here into an automated machine learning pipeline that is at the bleeding edge of what such systems can accomplish.
Sanyam Bhutani: H20.ai is working on many exciting projects, could you tell us more about your role at H2o.ai?
Dr. Bojan Tunguz: I work with the engineering team where I help with the development of DriverlessAI, as well as with our marketing, sales, and other outward-facing teams in their effort to promote our products, services, and the general ML approach and philosophy. I’ve been particularly excited about our recent educational initiative since it dovetails well with my former background in academia. I am also pretty involved with our efforts in the underwriting industry, where I bring my previous professional experience. H2O is a great organization for me to work at since it allows for the full spectrum of my talents and interests to be valued and utilized.
Sanyam Bhutani: You’ve had many amazing finishes on competitions.
Could you tell what was your favorite challenge?
Dr. Bojan Tunguz: My team, Home Aloan, recently finished 1st in the “Home Credit Default Risk,” the biggest Kaggle competition thus far. It was for so many reasons an incredible journey, and a dream come true for me. I write a bit more extensively about what made it so special in a discussion forum post that I wrote shortly after the competition ended.
Sanyam Bhutani: What kind of challenges do you look for today? How do you decide to enter a new competition?
Dr. Bojan Tunguz:
That’s easy — I enter all of them! :) However, most of the competitions I don’t put too much effort in. My favorite competitions are the NLP, image classification, and straightforward tabular data ones. Feature engineering is still not one of my strengths, so I don’t put too much effort into those kinds of competitions unless I can team up with someone who’s an expert feature engineer. I enjoy competitions where local improvements consistently lead to better leaderboard performance since there I can be pretty confident that my success will be proportional to my effort.
Sanyam Bhutani: Indeed I have noticed that as soon a competition launches you will soon tweet about a Top LB Submission.
What are your first steps and go to techniques when starting out on a new competition?
Dr. Bojan Tunguz: LOL, my tweets are usually just a joke. My first submission is just the sample submission. I tend to be a bit silly, and I “compete” with a few friends who will be the first one to get their name on the leaderboard.
My first “serious” steps in a competition involve some light EDA and building a simple first model or two, usually just a simple XGBoost, LightGBM, or both. Then I check how improvements in local CV correlate with improvements on LB, and how much of an impact ensembling has on the score. Depending on how all those experiments go, I’ll decide on the optimal strategy for the competition.
Sanyam Bhutani: For the readers and noobs like me who want to become better kagglers, what would be your best advice?
Dr. Bojan Tunguz: Don’t be afraid to fail and try to learn from your mistakes. Read the discussions in the forums, take a look at the best kernels, and try to improve upon them.
Sanyam Bhutani: Given the explosive growth rate of ML, How do you stay updated with the recent developments?
Dr. Bojan Tunguz: That’s a good question. I often say that the developments in ML are so blindingly rapid that I often feel like I have a permanent case of whiplash! Almost every week there is some new and exciting library or a framework, and I try to test and play with as many as my very limited time permits. I try to prioritize learning about tools and techniques that would have the greatest and most immediate impact on the projects that I am already working with or am familiar with.
Sanyam Bhutani: What developments in the field do you find to be the most exciting?
Dr. Bojan Tunguz: I feel there has been a very exciting explosion of NLP related tools and techniques over the last six months. I also feel that we’ve also experienced a lot of great advancements in terms of ML interpretability over the last year or so. These latter developments are not only helping us understand how the particular nonlinear ML algorithms work and what makes them effective, but could potentially pave the way for building even more advanced algorithms.
Sanyam Bhutani: What are your thoughts about Machine Learning as a field, do think its Overhyped?
Dr. Bojan Tunguz: Yes and no, and some parts more than others. There is no doubt that ML advances have been spectacular in recent years, and will likely continue on that upward trajectory for many years to come. Some of the most impressive advances have been in Deep Learning, but those techniques are the most effective in just a tiny subset of all problems to which ML can be applied. The biggest issue, as I see it, is that the application of ML in the industry is still in the very early stages. Most companies understand that it can help them in some ways, but are unsure of how. Many of them don’t have the infrastructure to take full advantage of what ML has to offer but are getting there. This all reminds me of the Internet in the 1990s: everyone was trying to do something about it, but most of those attempts were ill-conceived and led to a bubble that eventually burst. However, Internet use and applications kept growing exponentially, and now we are at the point where it’s quite literally everywhere and we can’t imagine life without it. I believe something similar will happen with ML.
Sanyam Bhutani: Before we conclude, any tips for the beginners who aspire to be like you someday but feel completely overwhelmed to even start competing?
Dr. Bojan Tunguz: I was that beginner just a few years ago, and felt probably the same way that most beginners feel. Two big “meta” pieces of advice that I would give all beginners is to give yourself time to develop, and don’t be afraid to fail. I would even go a step further: try to maximize the number of ways you fail. Try as many Kaggle competitions as you can, take as many online courses as you have the time for, or try to implement as many small projects as possible. You will most likely “fail” in one way or another at most of them, but make sure that you learn from all of those mistakes.
Another piece of advice that I would give you is to first focus on a few things that you do well, or like doing, and try to improve your skills in that niche. If you enjoy image classification problems, do more of those. If you are good at feature engineering and like coming up with new features, get even better at that. If implementing ML solution on the edge IoT devices is your thing, become an expert at it. However, don’t neglect your overall development as a Data Scientist or a Machine Learning practitioner, and keep adding other skills and tricks to your overall repertoire as you progress.
Sanyam Bhutani: Thank you so much for doing this interview.
Dr. Bojan Tunguz: You are welcome!
You can find me on twitter @bhutanisanyam1
Subscribe to my Newsletter for updates on my new posts and interviews with My Machine Learning heroes and Chai Time Data Science